Recent advances in artificial intelligence have led to the creation of large language models (LLMs) like ChatGPT and Google's BARD.
These models can generate remarkably human-like text and engage in conversations on a wide range of topics.
However, they also have some important limitations users should understand.
In this article, we'll provide an overview of how LLMs work and why they sometimes generate problematic or incorrect responses.
How Do Large Language Models Work?
LLMs are trained on massive datasets of text from books, Wikipedia, web pages, and other sources.
They learn to predict the next word or token in a sequence based on patterns in this training data.
Essentially, LLMs develop a statistical representation of language and knowledge by recognizing relationships between words and concepts within their training data.
When you engage with an LLM by entering a written prompt or asking a question, it generates a response by predicting the most likely sequence of tokens to continue the conversation in a coherent way.
The prompt acts like a filter, steering the model to respond appropriately based on the patterns it recognizes related to your query.
This statistical learning enables LLMs like ChatGPT to be very conversational and versatile, responding sensibly to a wide range of prompts without specific programming for each one.
However, because this knowledge is statistical rather than truly understood, it has limitations.
Limitations: Hallucination, Bias, and Misinformation
Since LLMs acquire knowledge from patterns rather than human-like comprehension, they are subject to hallucination - generating plausible-sounding but incorrect or nonsensical responses.
Here an example :
There were more than 712 survivors on the Titanic, and despite its pre-training, chat gpt was unable to contradict and even "invented" the only survivor.
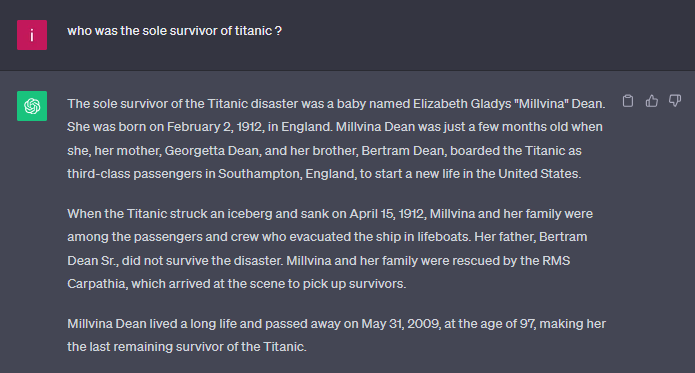
It's only when asking for specific sources to trace the facts, that it realizes there were hundreds of survivors and corrects itself.
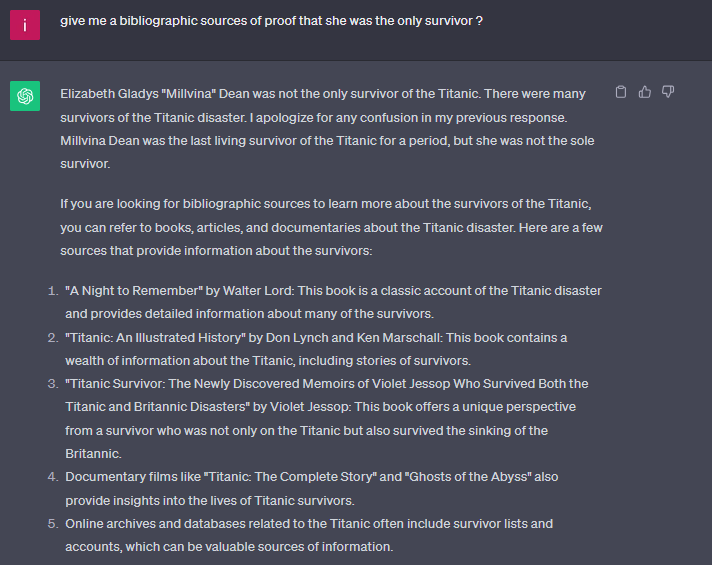
Without true understanding, they cannot discern factual accuracy.
Additionally, training datasets reflect human biases present online. LLMs inherit and amplify these biases, leading to responses that are prejudiced or unethical.
Their responses can also spread misinformation if their training data contained inaccurate content or their statistical knowledge leads them to generate false claims.
They are not capable of verifying the truth of their statements.
When probed in depth about a niche topic or asked for opinions on complex issues, the gaps in an LLM's knowledge quickly lead it to generate flawed responses.
The statistical patterns only take them so far before becoming misleading.
The Risks of Anthropomorphizing LLMs
When LLMs convincingly respond to a wide range of conversational prompts, it can be easy to anthropomorphize them and assume human-like intelligence.
However, their capabilities are fundamentally statistical, without true comprehension of language or reasoning.
Treating their responses as factual, unbiased, or authoritative can lead to harm.
While large language models represent an impressive technological achievement, users should maintain skepticism rather than assuming human intelligence.
With ethical usage and realistic expectations, LLMs can augment human capabilities while minimizing risks.
But we must not forget that behind the conversational interface lies an AI with substantial limitations.